Using Artificial Intelligence to Help Smallholder Farmers Combat Climate Change
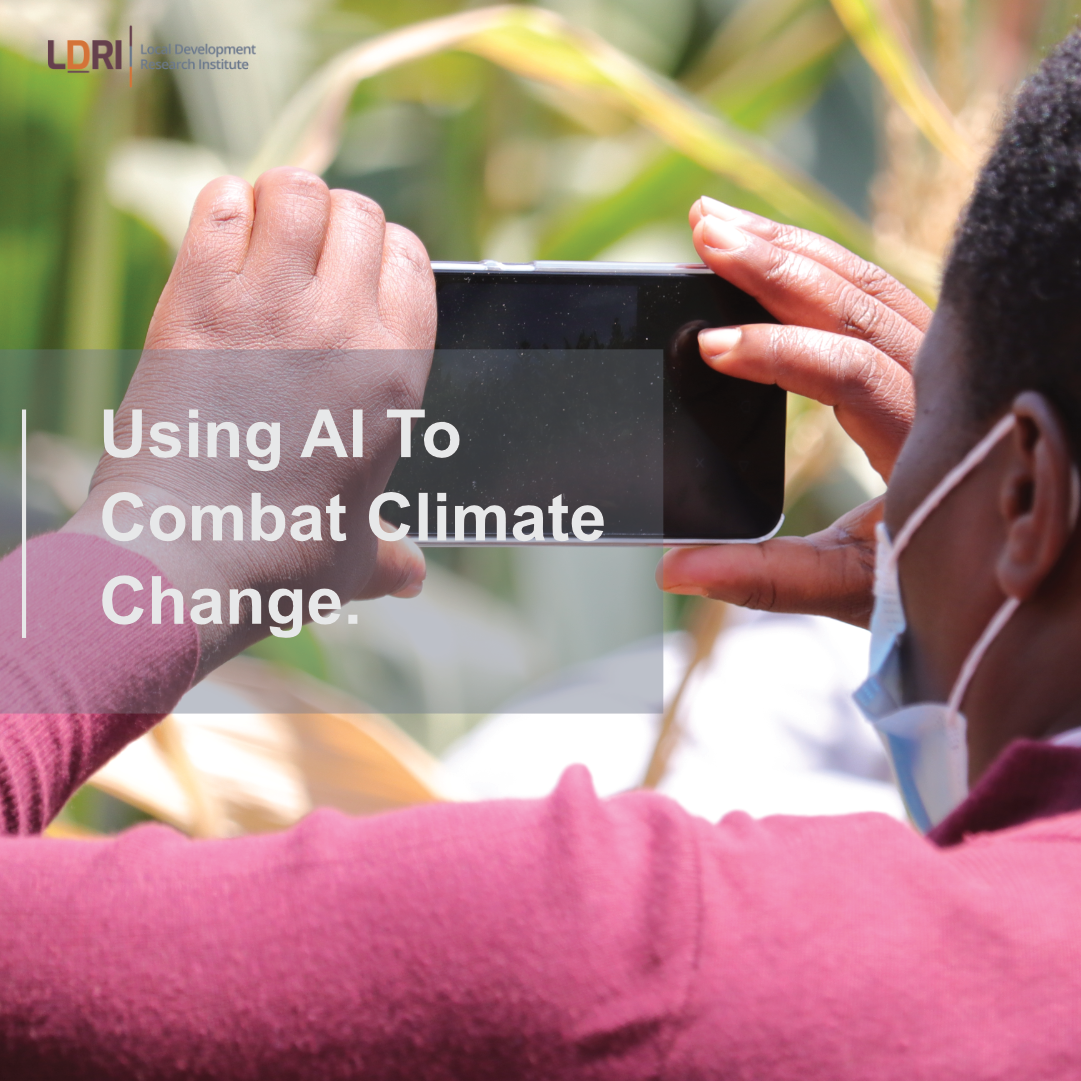
Over the last few years, climate-smart agriculture(CSA) has been fronted as a way to help smallholder farmers cope with the effects of climate change and remain food and nutrition secure. In Sub-Saharan Africa, up to 80% of the population is heavily dependent on rain-fed agriculture by smallholder farmers. In most cases, these farmers are reliant on intuition and years of experience to plant their crops and feed their livestock. But as climate change worsens, it’s becoming harder to rely on intuition and experience alone.
As the majority of crop production in sub-Saharan Africa is by subsistence farming, CSA interventions need to be made at both the policy and household levels. However, to achieve the goal to be sustainably food secure, we need to maximize and improve farm productivity. Farmers require support in the form of access to extension services and early warning systems, access to quality inputs and seeds that are more resilient to climate change, and other agronomic best practices. Additionally, access to accurate data on each crop production cycle supports data-driven decision-making by agriculture and nutrition policymakers.
With advancements in technology, governments and other stakeholders are increasingly turning towards AI to support decision-making in the agriculture and nutrition sectors. In the sub-Saharan African context, however, these solutions cannot be applied in a straightforward manner for a variety of reasons.
Smallholder agriculture is dominant with most farms ranging from 0.5 to 3ha in size. Additionally, most smallholder farmers practice intercropping and mixed land use and areas under cultivation of specific crops are not always clearly demarcated. These challenges make monitoring crop health and estimating yield per farm difficult when using big data tools such as satellite imagery, especially when ground-truth data is not available. Moreover, it becomes difficult to develop an intelligent and automated system to provide predictive insights based on observation of each crop cycle and an early warning of crop failure at scale.
Building a Localized Early Warning System
Several early warning systems have been developed and implemented across Sub-Saharan Africa over the past decades. Ranging from conflict management to drought to crop pests, these early warning mechanisms have provided signals to policymakers that have enabled them to act fast to mitigate the negative impacts of these threats. However, many of these still require the production of fairly complex reports as outputs for use by policymakers for decision-making. Few, if any, are designed to provide this information inaccessible and contextually relevant ways to smallholder farmers and those who support them on the ground.
In 2019, the Local Development Research Institute rolled out a project to provide smallholder farmers in Embu and Kiambu counties of Kenya with information on the most appropriate hybrid varieties of maize to grow and the right agronomic practices to adopt to increase the chances of a good harvest. Through this project, the information needed by farmers and the public sector’s agricultural extension staff has been made available and accessible through peer farmer educators and mobile phone-based services.
Currently, we work with a group of 300,000+ farmers and for each crop cycle, we continue to collect data using mobile phones data on the variety of maize seeds planted, the number of seeds planted and the harvest in bags obtained per season. We have also been issuing them with sample hybrid varieties to test each season, and are starting to note differences in yield between the sample varieties and their usual varieties. However, due to uncertainties around the approximations of land boundaries and intercropping, we are yet to accurately predict or calculate the area under cultivation of the various maize varieties from all farms supported to effectively assess the impact of cultivating the climate-smart hybrid varieties. We can also not send out information on mitigating measures to farmers in good time to respond to potential imminent crop failure. This provides a unique opportunity for remote sensing and AI to support widespread data collection and analysis of crop yield estimates and predicted crop failure at scale and avail synthesized results and information back to the farmer through a more open and sustainable approach. This level of granular intelligence for farmers is not available from other early warning systems.
With the support of GIZ, throughout 2022, LDRI is developing an AI early warning system that monitors farming activities and supports precision agriculture practices using hyperlocal geo-referenced data and high-resolution satellite imagery powered by mobile, satellite, and remote sensing technology.
The project is part-research, part-action oriented. We aim to not only study just how such an AI-based intervention could improve food and nutrition security within the communities we work with but also document best practices of co-designing and co-developing this AI within these communities in a responsible manner.
Our Theory of Change
The early warning system is part of a broader offering LDRI will be providing to Kenya’s county governments under our Extension Support Program. As part of this work, LDRI has implemented a technology platform known as ZydiPlus that can disseminate information on good agricultural practices, availability of appropriate inputs, and information on relevant events happening in their areas.
When subsistence farmers are able to receive contextually relevant information about their crop health, conditioned on weather predictions, specific soil conditions, and can implement in a timely fashion the right practices such as irrigation or selection of alternative crops to adapt to prevailing factors, they are able to maximize their farm productivity, producing enough to remain food secure and commercialize the excess harvest. Similarly, when policymakers have access to timely data on crop performance or imminent crop failure at a granular level and can accurately estimate the area under cultivation, they are able to plan and target specific interventions for requisite inputs and subsidies needed in the area as well as target social security programs in the case of pending crop failure.
The datasets and AI system we aim to produce can be to monitor crop health and predict crop yields from various varieties and with high accuracy predict area under cultivation and estimated yields, necessary metrics to monitor to maintain food and nutrition security. We shall refine this theory of change as the project progresses.
In the next blog, we will discuss more in-depth our approach to building this AI and the progress made so far.